- To what extent will AI change gaming in the next five years?
- To what extent is the search for talent already supported by artificial intelligence?
- Would an AI recognize and take into account when young people are particularly developed or lagging behind their biological age?
- Can amateur clubs also apply this relatively easily?
- To what extent do AI systems support rehabilitation?
- Would an AI also tell me when I need to take a break?
- What does the use of AI in learning/improving movement techniques look like?
- Is it really possible for a camera to record a game from 1000 perspectives and then spit out a tactic?
Coaches have been working with a wealth of training data for a long time in the world of sport, especially soccer. First division teams, for example, were the first to integrate new technologies, as measuring and evaluating physiological parameters has always been part of the job description of their fitness coaches. During training and matches, first without and later with the ball, the coaching team can easily record the speed, acceleration and distance covered by players. Today, this data is also analysed with the help of artificial intelligence (AI). Coaches integrate the insights gained into their training plans, derive load profiles and manage the sessions. AI can now also be used to plan and analyse complex training content with a ball.
Daniel Memmert is involved in the scientific aspects of such developments. Memmert is Professor and Managing Director of the Institute for Training Science and Sports Informatics at the German Sport University Cologne. He has published numerous (specialist) publications, for example on data analysis in soccer, and conducts research for the German Football Association and the German Football League, among others. For ISPO.com, he gives a forecast of how far AI will change sport and shows what is possible today in top-class sport and will soon be possible in recreational sport with AI.
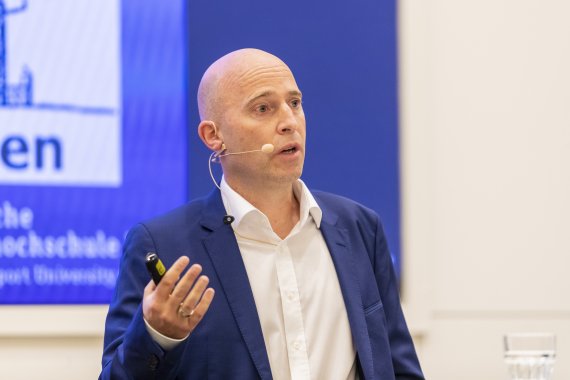
Massive. The main factor will be scouting. Only around ten percent of professional clubs use data scouting at the moment. This will explode in the coming years. There are many leagues, such as the Brazilian and Argentinian leagues, that do not yet collect satisfactory tracking or positional data. At some point, all leagues will have this data. For example, you will then be able to click on a list of players with certain metrics that the respective club considers important. You can then watch the ten best players from this list again live or scout them via video. Some clubs are already employing fewer scouts, but conventional player monitoring is still predominant at present. In the coming years, data scouting will also extend to the U17 and U19 youth divisions in order to be able to search, list, analyse and evaluate players more quickly, efficiently and effectively according to certain KPIs.
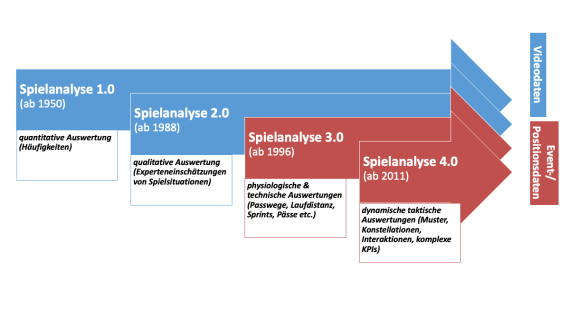
With our self-developed and validated tool, we use historical data from the Bundesliga clubs and calculate, for example, the probabilities of which players from the U12s should already move to the U14s. Or what market value and how many professional minutes players will have later on. The basis is always the historical data, which gives us an estimate for the future.
We would use other measures. There is BioBending, for example. This involves grouping and assessing players in training and competitions according to their biological stage of development rather than their calendar age in order to avoid the problem of the "relative age effect". At the same time, it is statistically the case that players born late in the year collect more professional minutes at some youth academies than those born between January and March. This is known as the "reversed relative age effect". As the younger players are constantly playing with stronger/larger players and have to assert themselves, this can also result in advantages. In fact, we are also starting to work with AI here. We have already developed prediction models to incorporate the relative age effect.
Of course, this is very time-consuming overall. However, there are increasingly cheaper camera systems that provide video data from which tracking data can be extracted. This has been in use for around five years. In the future, these options will soon be affordable for many amateur clubs, as the sensors will no longer cost as much. This creates a business model for many technology companies to work with smaller clubs.
The technical term for this is "injury prediction". There are studies that show the probability of players getting injured if they are not spared. You need medical data for this, which is why it is very difficult to publish something like this or make it transparent. This is where we still need the most research. We want to gain a better understanding of the history of injuries that lead to players being spared. At the same time, we are investigating in which situations in a match - for example in which type of tackle - the risk of injury is particularly high. Such questions can be answered very well with AI based on large amounts of historical data. We are currently trying to find sponsors for such projects.
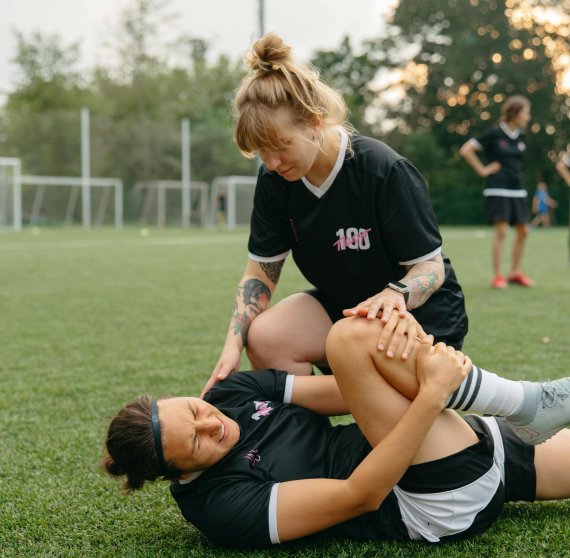
Yes, of course. As soon as historical data or even comparative data within groups is available, whereby historical data from an athlete makes more sense, then this AI uses this information to control the training accordingly.
The AI attempts to classify the performance data and, based on the available data from the past, it knows which training area the athlete is in.
In my eyes, movement techniques are highly individual. I reject the idea of generating standards. There is no such thing as the golden standard technique; instead, many different techniques lead to top performances due to biomechanical and anthropometric conditions. For example, the top ten tennis players in the world have relatively different techniques for the serve, backhand and forehand. As there is no ideal standard technique, the use of AI is much more complex here. A lot of time would have to be invested to achieve meaningful results. We are at the very beginning.
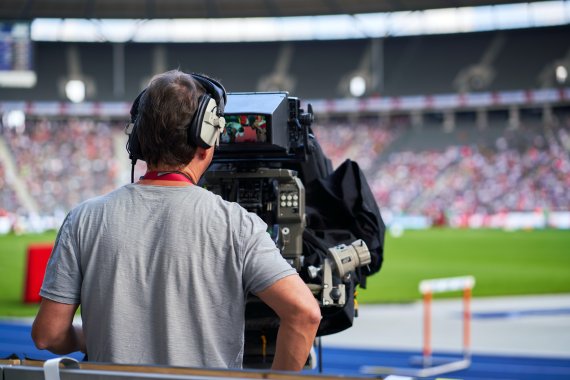
Yes, yes, that's exactly how it works. For example, we have the data from the German Bundesliga. This allows us to read out the spatial control of the players, the entire team, the pressing values, the opponents overplayed and many other tactical variables in fractions of a second. As the sensor data from the ball and players is now highly reliable, everything is tracked very accurately. This means we have first-class data and very good AI-supported analyses.
- Awards
- Mountain sports
- Bike
- Fitness
- Health
- ISPO Munich
- Running
- Brands
- Sustainability
- Olympia
- OutDoor
- Promotion
- Sports Business
- Textrends
- Triathlon
- Water sports
- Winter sports
- eSports
- SportsTech
- OutDoor by ISPO
- Heroes
- Transformation
- Sport Fashion
- Urban Culture
- Challenges of a CEO
- Trade fairs
- Sports
- Find the Balance
- Product reviews
- Newsletter exclusive area
- Magazine